Penyakit COVID-19 yang disebabkan oleh virus SARS-CoV-2 dapat ditularkan melalui aerosol. Aerosol berukuran kecil dapat masuk langsung sampai ke alveoli di paru-paru
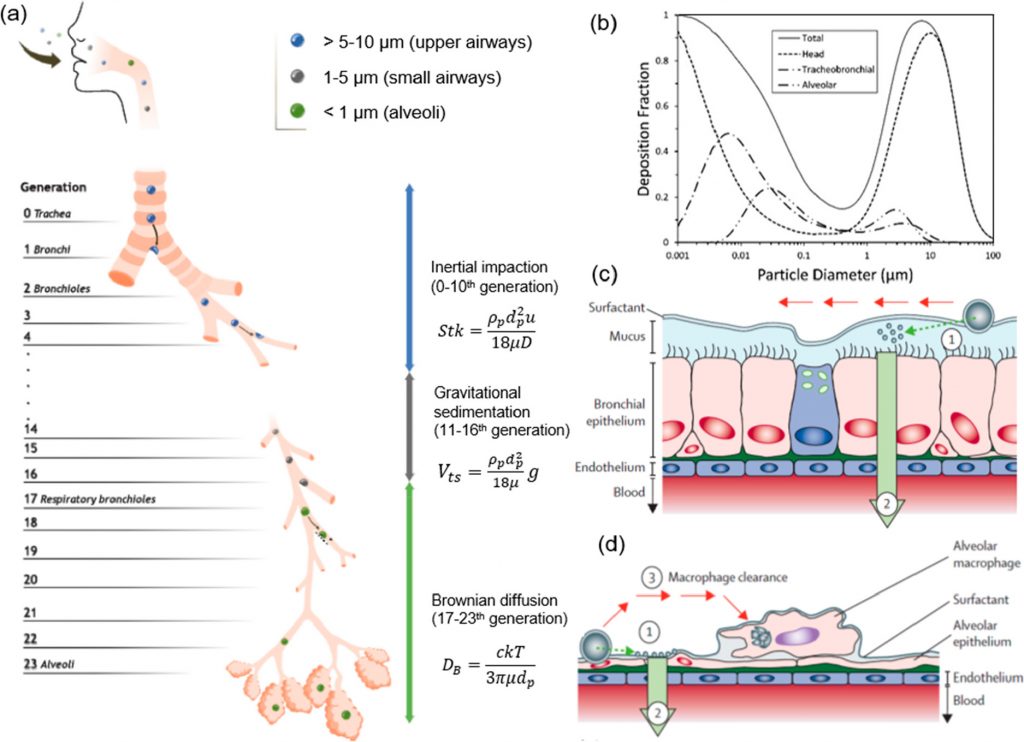
Penyakit COVID-19 yang disebabkan oleh virus SARS-CoV-2 dapat ditularkan melalui aerosol. Aerosol berukuran kecil dapat masuk langsung sampai ke alveoli di paru-paru
Article from blog.intent.to
Probabilistic Machine Learning: an Introduction by Kevin P. Murphy
URL: https://probml.github.io/pml-book/book1.html
Sumber: https://globalsolaratlas.info
Berikut ini peta potensi tenaga listrik (Specific photovoltaic power output) untuk sedunia
Berikut ini potensi tenaga listrik (Specific photovoltaic power output) untuk Indonesia.
Alat pendeteksi COVID-19 buatan para ahli di Universitas Gadjah Mada (UGM) yaitu GeNose, akhirnya mengantongi izin edar dari Kemenkes dan siap dipasarkan.
“Alhamdulillah berkat doa & dukungan luar biasa dari banyak pihak GeNose C19 secara resmi mendapatkan izin edar untuk mulai dapat pengakuan oleh regulator (Kemenkes) dalam membantu penanganan COVID-19 melalui skrining cepat,” kata Kuwat Triyana, Ketua tim pengembangan GeNose.
Lebih lanjut, Kuwat menjelaskan kalau GeNose punya cara yang beda untuk mendeteksi keberadaan virus SARS-CoV-2 jika dibandingkan dengan metode swab test PCR.
Dijelaskan Kuwat, GeNose hanya membutuhkan embusan nafas yang ditiupkan ke alat dan hasil akan keluar dalam waktu dua menit. Terus gimana dengan akurasinya?
Menristek/Kepala Badan Riset Inovasi Nasional (BRIN) Bambang Brodjonegoro beberapa waktu lalu mengungkapkan melalui uji coba tahap pertama di rumah sakit, tes COVID-19 GeNose menunjukkan tingkat akurasi 97 persen.
Dengan biaya tiap tes yang rencananya hanya dipatok pada kisaran Rp15-25 ribu saja, Kuwat menyebut saat ini telah ada 100 unit GeNose yang diproduksi pihaknya pada tahap pertama dan akan segera didistribusikan.
Meski belum banyak, dia berharap 100 alat ini setidaknya mampu melakukan tes terhadap 12 ribu orang sehari.
Paper ilmiah alat ini dapat diakses di artikel “Intelligent Mobile Electronic Nose System Comprising a Hybrid Polymer-Functionalized Quartz Crystal Microbalance Sensor Array“
Pengujian klinis alat ini sedang dilakukan. Catatannya ada di artikel “Genosvid Diagnostic Test for Early Detection of COVID-19”
Berikut ini pengumuman konferensi pers tentang GeNose UGM tersebut.
Berapakah tingkat herd immunity yang diperlukan untuk mengatasi Covid19?
Berapakah orang yang meninggal jika Indonesia menerapkah herd immunity tanpa vaksin?
Penduduk Indonesia : 267 juta (https://nasional.kompas.com/read/2020/08/12/15261351/data-kependudukan-2020-penduduk-indonesia-268583016-jiwa)
Anggap saja pakai batas bawah WHO: 60%
Total terinfeksi: = 267 juta x 60% = 160.2 juta
Tingkat kematian Covid19 di Indonesia 3,4 % (https://health.detik.com/berita-detikhealth/d-5282583/who-angka-kematian-covid-19-di-indonesia-lebih-tinggi-dari-dunia)
Jumlah kematian = 160.2 x 3.4% = 5.4468 juta
Saat ini jumlah orang meninggal karena Covid19 adalah 18000 orang (https://nasional.kompas.com/read/2020/12/10/11375561/indonesia-catat-kematian-covid-19-tertinggi-dan-992-persen-wilayah-terpapar)
Jadi jumlah korban meninggal total sekurangnya 300x jumlah meninggal sekarang.
Tingkat kematian tersebut dengan asumsi fasilitas kesehatan mencukupi. Jika rumah sakit overload, maka persentase warga yang meninggal akan lebih tinggi dari 3,4%.
Jadi jumlah korban yang meninggal adalah 5,4468 juta.
Berikut ini kelemahan strategi Herd Immunity tanpa vaksin
Berikut ini studi yang menunjukkan adanya kemungkinan terjadinya transmisi virus melalui saluran pembuangan air di sebuah apartemen.
Lokasi: sebuah apartemen di Guangzhou, China
Waktu: Januari ~ Februari 2020
Salah satu tindakan yang dilakukan orang adalah menutup saluran pembuangan air, supaya tidak ada aerosol masuk melalui saluran air [ref].
Ada kasus terjadinya penularan COVID19 di sebuah apartement. Kemungkinan transmisi virus tersebut adalah melalui saluran ventilasi.
Lokasi: Seoul, Korea Selatan
Waktu: Agustus 2020
Web server Apache akan menjadi lambat / hang jika diakses oleh terlalu banyak client, sedangkan memori sudah habis. Akan terjadi permintaan memory swap yang besar sehingga semuanya akan semakin lambat. Pada akhirnya koneksi akan terputus. Untuk membatasi jumlah client, dapat digunakan setting “MaxRequestWorkers”.
Berikut ini salah satu contoh setting untuk server yang memorynya sedikit.
<IfModule mpm_worker_module>
ServerLimit 5
StartServers 2
MaxRequestWorkers 20
MinSpareThreads 5
MaxSpareThreads 5
ThreadsPerChild 5
</IfModule>
Perangkat lunak jaringan dapat diimplementasikan dengan beberapa cara. Pertama dapat menggunakan arsitektur monolitik, di mana semua fungsi digabung di sebuah modul besar. Cara kedua adalah dengan memisahkan fungsi-fungsi tersebut menjadi beberapa modul, dengan setiap modul dapat berinteraksi dengan modul lain. Cara ketiga adalah dengan memisahkan fungsi-fungsi tersebut menjadi beberapa modul, dan modul disusun dengan lapisan (layer) dengan suatu hirarki tertentu, sehingga 1 modul hanya dapat berkomunikasi dengan 1 modul di atasnya.
Berikut ini struktur lapisan/ layering pada sistem komunikasi pada 2 buah host/perangkat.
Penomoran lapisan dimulai dari bawah. Layer paling bawah adalah medium fisik, dapat berupa kabel tembaga, fiber optic, wireless dan sebagainya.
Setiap perangkat memiliki susunan layer/lapisan yang sama. Antara setiap layer terdapat interface (antar muka) yang terdefinisi dengan baik. Layer 5 hanya berkomunikasi dengan layer 4. jadi layer 5 tidak tahu dan tidak perlu tahu teknis detail di layer 3,2 dan fisik. Layer 5 di host 1 seakan-akan berkomunikasi dengan layer 5 di host 2 , walaupun sebenarnya data yang dikirim akan melewali layer-layer di bawahnya.
Setiap layer akan memproses header/trailer pada data yang melaluinya. Pada waktu data dikirim, header atau trailer akan ditambahkan pada data yang dikirim. Pada proses penerimaan, header atau trailer tersebut dipotong, tidak diteruskan ke layer di atasnya.
Berikut ini adalah contoh aliran data dari suatu mesin sumber (source machine) ke mesin tujuan (destination machine). Data M di source dibuat di layer 5. Data ini kemudian dikirimkan ke layer 4. oleh layer 4, data ini ditambahi fitur-fitur tertentu, sesuai dengan tugas layer 4 tersebut. Misalnya adalah dengan ditambah error detection. Fitur error detection ini perlu tambahan data, misal untuk pengecekan parity atau CRC (cyclic redundancy check). data untuk error detection dan data-data lain terkait layer 4 ditambahkan sebagai H4 (header layer 4). H4+M ini kemudian dikirimkan ke layer berikutnya: layer 3. Di layer 3 proses serupa dilakukan, dan layer 3 menambahkan H3, sehingga sekarang data yang dikirim menjadi H3+H4+M. Demikian juga diteruskan di layer 2.
Pada akhirnya data yang betul-betul dikirim secara fisik melalui medium fisik adalah H2+H3+H4+M, dan data ini diterima di destination machine.
pada proses penerimaan di destination machine, proses sebaliknya dilakukan, yaitu header di setiap layer diproses sesuai dengan fitur pada layer tersebut, dan header tersebut dibuang. data yang sudah dibuang headernya dikirim ke layer di atasnya. Jadi di sini misalnya pada layer 2 akan memproses H2 dan membuangnya. sehingga layer 3 akan menerima H3+H4+M. Demikian seterusnya di layer 3.
Pada layer 4, H4 diproses. Jika misalnya di pengiriman H4 berisi kode error detection, maka error detection akan dicek di layer 4 ini. jika hasilnya sesuai, maka data M akan dikirimkan ke layer 5 di destination. jika hasilnya tidak sesuai, maka data tersebut akan dibuang atau diminta untuk dikirim ulang dari source machine, tergantung mekanisme protokol yang dipilih.
Salah satu layanan dari layer komunikasi adalah layer atas tidak perlu tahu dan dibatasi oleh kondisi layer bawah. Secara sistem engineering hal ini disebut sebagai ‘abstraksi’.
Sebagai contoh adalah masalah ukuran data. Dari sisi pemakai atau pembuat aplikasi tentunya hanya ingin mengirim data dengan ukuran berapapun, tidak ingin direpotkan dengan pembatasan ukuran data. sedangkan di sisi fisik hanya mampu mengirim data dengan ukuran data.
Misal Wifi maksimum 2346 byte, dan kabel ethernet maksimuk 1536 byte. akan repot bagi user ketika ingin browsing ke suatu situs, tapi harus memilih ukuran data maksimum 2346 byte atau 1536, tergantung jaringannya sedang menggunakan kabel, atau tethering via wireless ke smartphone.
Dalam contoh ini layer 3 tidak sanggup mengirim data M secara utuh, sehingga data harus dipisahkan menjadi 2 segmen, yaitu M1 dan M2, kemudian masing=masing bagian ini dikirim sebagai 2 pesan terpisah: M1 dan M2 ke layer 3. Layer 3 H4+M , namun memecahnya menjadi 2 bagian: H3+H4+M1 dan H3+M2. Layer 2 meneruskan keduanya tanpa tahu bahwa M1 dan M2 sebenarnya berhubungan. layer fisik juga hanya meneruskannya saja.
Di penerima, kedua pesan yang mengandung M1 dan M2 ini diterima sampai ke layer 3 seperti biasa. Di layer 3 kedua pesan M1 dan M2 digabung, kemudian baru dikirim ke layer 4. Dalam prakteknya dapat saja kedua pesan M1 dan M2 tersebut sampai tidak berurutan atau ada yang hilang, sehingga layer 3 perlu melakukan tindakan-tindakan untuk mengatasinya.
Aliran Data Dengan Perantara
Gambar sebelumnya menunjukkan aliran data antara 2 perangkat saja. Dalam prakteknya, jaringan melibatkan 1 atau lebih perangkat penghubung (connecting device) sebagai perantara, seperti switch dan router.
Pada gambar ini, terdapat sumber (source) A yang mengirimkan data ke tujuan (destination) B.
Model jaringan yang dipakai di sini adalah TCP/IP, dengan layernya adalah pysical, datalink, network, transport dan application.
Di antara keduanya terdapat switch dan router. switch di sini fungsinya menghubungkan secara fisik antara source ke router, dan router ke destination. Di dalam switch dan router terdapat juga layer-layer jaringan.
Pada switch, layer yang diurus adalah fisik sampai ke datalink.
Pada router, layer yang diurus adalah fisik sampai ke network. Pada source dan destination, layernya ada lengkap, dari physical sampai application.
Perangkat lunak jaringan dapat diimplementasikan dengan beberapa cara. Pertama dapat menggunakan arsitektur monolitik, di mana semua fungsi digabung di sebuah modul besar. Cara kedua adalah dengan memisahkan fungsi-fungsi tersebut menjadi beberapa modul, dengan setiap modul dapat berinteraksi dengan modul lain. Cara ketiga adalah dengan memisahkan fungsi-fungsi tersebut menjadi beberapa modul, dan modul disusun dengan lapisan (layer) dengan suatu hirarki tertentu, sehingga 1 modul hanya dapat berkomunikasi dengan 1 modul di atasnya.
Berikut ini struktur lapisan/ layering pada sistem komunikasi pada 2 buah host/perangkat.
Penomoran lapisan dimulai dari bawah. Layer paling bawah adalah medium fisik, dapat berupa kabel tembaga, fiber optic, wireless dan sebagainya.
Setiap perangkat memiliki susunan layer/lapisan yang sama. Antara setiap layer terdapat interface (antar muka) yang terdefinisi dengan baik. Layer 5 hanya berkomunikasi dengan layer 4. jadi layer 5 tidak tahu dan tidak perlu tahu teknis detail di layer 3,2 dan fisik. Layer 5 di host 1 seakan-akan berkomunikasi dengan layer 5 di host 2 , walaupun sebenarnya data yang dikirim akan melewali layer-layer di bawahnya.
Setiap layer akan memproses header/trailer pada data yang melaluinya. Pada waktu data dikirim, header atau trailer akan ditambahkan pada data yang dikirim. Pada proses penerimaan, header atau trailer tersebut dipotong, tidak diteruskan ke layer di atasnya.
Berikut ini adalah contoh aliran data dari suatu mesin sumber (source machine) ke mesin tujuan (destination machine). Data M di source dibuat di layer 5. Data ini kemudian dikirimkan ke layer 4. oleh layer 4, data ini ditambahi fitur-fitur tertentu, sesuai dengan tugas layer 4 tersebut. Misalnya adalah dengan ditambah error detection. Fitur error detection ini perlu tambahan data, misal untuk pengecekan parity atau CRC (cyclic redundancy check). data untuk error detection dan data-data lain terkait layer 4 ditambahkan sebagai H4 (header layer 4). H4+M ini kemudian dikirimkan ke layer berikutnya: layer 3. Di layer 3 proses serupa dilakukan, dan layer 3 menambahkan H3, sehingga sekarang data yang dikirim menjadi H3+H4+M. Demikian juga diteruskan di layer 2.
Pada akhirnya data yang betul-betul dikirim secara fisik melalui medium fisik adalah H2+H3+H4+M, dan data ini diterima di destination machine.
pada proses penerimaan di destination machine, proses sebaliknya dilakukan, yaitu header di setiap layer diproses sesuai dengan fitur pada layer tersebut, dan header tersebut dibuang. data yang sudah dibuang headernya dikirim ke layer di atasnya. Jadi di sini misalnya pada layer 2 akan memproses H2 dan membuangnya. sehingga layer 3 akan menerima H3+H4+M. Demikian seterusnya di layer 3.
Pada layer 4, H4 diproses. Jika misalnya di pengiriman H4 berisi kode error detection, maka error detection akan dicek di layer 4 ini. jika hasilnya sesuai, maka data M akan dikirimkan ke layer 5 di destination. jika hasilnya tidak sesuai, maka data tersebut akan dibuang atau diminta untuk dikirim ulang dari source machine, tergantung mekanisme protokol yang dipilih.
Salah satu layanan dari layer komunikasi adalah layer atas tidak perlu tahu dan dibatasi oleh kondisi layer bawah. Secara sistem engineering hal ini disebut sebagai ‘abstraksi’.
Sebagai contoh adalah masalah ukuran data. Dari sisi pemakai atau pembuat aplikasi tentunya hanya ingin mengirim data dengan ukuran berapapun, tidak ingin direpotkan dengan pembatasan ukuran data. sedangkan di sisi fisik hanya mampu mengirim data dengan ukuran data.
Misal Wifi maksimum 2346 byte, dan kabel ethernet maksimuk 1536 byte. akan repot bagi user ketika ingin browsing ke suatu situs, tapi harus memilih ukuran data maksimum 2346 byte atau 1536, tergantung jaringannya sedang menggunakan kabel, atau tethering via wireless ke smartphone.
Dalam contoh ini layer 3 tidak sanggup mengirim data M secara utuh, sehingga data harus dipisahkan menjadi 2 segmen, yaitu M1 dan M2, kemudian masing=masing bagian ini dikirim sebagai 2 pesan terpisah: M1 dan M2 ke layer 3. Layer 3 H4+M , namun memecahnya menjadi 2 bagian: H3+H4+M1 dan H3+M2. Layer 2 meneruskan keduanya tanpa tahu bahwa M1 dan M2 sebenarnya berhubungan. layer fisik juga hanya meneruskannya saja.
Di penerima, kedua pesan yang mengandung M1 dan M2 ini diterima sampai ke layer 3 seperti biasa. Di layer 3 kedua pesan M1 dan M2 digabung, kemudian baru dikirim ke layer 4. Dalam prakteknya dapat saja kedua pesan M1 dan M2 tersebut sampai tidak berurutan atau ada yang hilang, sehingga layer 3 perlu melakukan tindakan-tindakan untuk mengatasinya.
Gambar sebelumnya menunjukkan aliran data antara 2 perangkat saja. Dalam prakteknya, jaringan melibatkan 1 atau lebih perangkat penghubung (connecting device) sebagai perantara, seperti switch dan router.
Pada gambar ini, terdapat sumber (source) A yang mengirimkan data ke tujuan (destination) B.
Model jaringan yang dipakai di sini adalah TCP/IP, dengan layernya adalah pysical, datalink, network, transport dan application.
Di antara keduanya terdapat switch dan router. switch di sini fungsinya menghubungkan secara fisik antara source ke router, dan router ke destination. Di dalam switch dan router terdapat juga layer-layer jaringan.
Pada switch, layer yang diurus adalah fisik sampai ke datalink.
Pada router, layer yang diurus adalah fisik sampai ke network. Pada source dan destination, layernya ada lengkap, dari physical sampai application.
Pada komunikasi data melalui layer-layer tersebut, terjadi proses penambahan header dan pemotongan header. Proses penambahan header disebut juga sebagai proses encapsulation. Pada proses ini data dari layer di atasnya seakan-akan ‘dibungkus’ dengan header yang ditambahkan. Pada bagian penerimaan data, terjadi proses pemotongan header. Proses ini disebut sebagai ‘decapsulation’. Pada proses ini seakan-akan ‘bungkus’ dari layer tersebut dibuka, kemudian isi dari ‘bungkus’ tersebut dikirmkan ke layer di atasnya.