Scikit-learn has just introduced simple API for machine learning visualizations. Here are a few visualization samples:
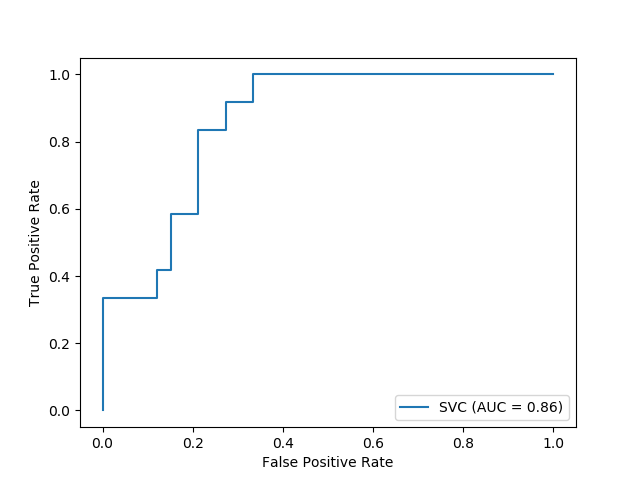
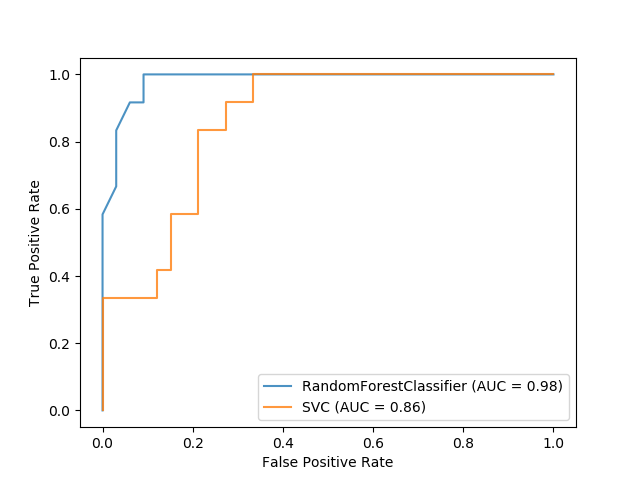
Artikel Saintek Umum
Scikit-learn has just introduced simple API for machine learning visualizations. Here are a few visualization samples:
Kumpulan artikel-artikel tentang “Making Indonesia 4.0”.
Dokumen Resmi Making Indonesia 4.0
Artikel Berita tentang Making Indonesia 4.0
List of object detection progress:
Reference: https://deeplearning.mit.edu/
Berikut ini siaran pers bersama, yang nampaknya pengumuman resmi paling lengkap sejauh ini tentang bencana tsunami di Selat Sunda.
Siaran Pers Bersama dari lembaga-lembaga berikut:
Sumber data ini adalah twitter dari BIG (Badan Informasi Geospasial) dalam format gambar JPG. Sejauh ini belum didapatkan versi PDF ataupun versi website.
Sumber:
Data korban dan kerusakan ini diperoleh dari twitter pak Sutopo Purwo Nugroho (Humas BNPB) di alamat https://twitter.com/Sutopo_PN/status/1077092955389812736
Sejauh ini belum ada gambar resmi dari situs Balai Nasional Penanggulanan Bencana (BNPB)
![]() |
Keras logo |
There are several kind of image classification:
Image generation method for training
Various models for training (built on model)
Keras built in models usually have pre-trained weight on Imagenet, which significantly speeds up training, but those weights are only available for some image sizes.
There are two techniques to feed image files for prediction in Keras:
keras.preprocessing.image.flow_from_directory()
keras.preprocessing.image.flow()
This tutorial shows how to do multiclass image classification with Keras, using keras.preprocessing.image.flow_from_directory() to feed the image files for training and prediction.
![]() |
||
Plant Seedlings Classification dataset |
Here is the directory structure after previous steps:
import tensorflow as tf
import keras as keras
import os
from keras.layers import Flatten, Dense, AveragePooling2D, GlobalAveragePooling2D
from keras.models import Model
from keras.optimizers import RMSprop, SGD
from keras.callbacks import ModelCheckpoint
from keras.callbacks import EarlyStopping
from keras.preprocessing.image import ImageDataGenerator
from keras.callbacks import CSVLogger
from keras.layers.normalization import BatchNormalization
import numpy as np
from keras.models import load_model
from pathlib import Path
import shutil
Create directories for training and validation set. A little bit complicated, since flow_from_directory() required that each class has it’s own directory.
#making training & validation directories
import pathlib
session='simpleNASNet'
classnames=['Black-grass','Charlock','Cleavers','Common Chickweed','Common wheat','Fat Hen','Loose Silky-bent','Maize','Scentless Mayweed','Shepherds Purse','Small-flowered Cranesbill','Sugar beet']
train_dir="../"+session+"/train"
valid_dir="../"+session+"/valid"
for dirname in classnames:
# print(dirname)
fulldirname=train_dir+'/'+dirname
print(fulldirname)
pathlib.Path(fulldirname).mkdir(parents=True, exist_ok=True)
fulldirname=valid_dir+'/'+dirname
print(fulldirname)
pathlib.Path(fulldirname).mkdir(parents=True, exist_ok=True)
Split training data between training set and validation set. Usual 80%-20% split is used.
#copy image files, split 80% training- 20% validation
counter=0
for root, dirs, files in os.walk(original_data_dir):
for file in files:
fullfilename = os.path.join(root, file)
basename=os.path.basename(fullfilename)
#detect image classification from directory name
split1=os.path.split(fullfilename)
split2=os.path.split(split1[0])
classname=str(split2[1])#classname for this particular file
if((counter%5)==0): #copy validation
dst_filename=valid_dir+"/"+classname+"/"+basename
shutil.copyfile(fullfilename,dst_filename)
else: #copy training
dst_filename=train_dir+"/"+classname+"/"+basename
shutil.copyfile(fullfilename,dst_filename)
counter=counter+1
Prepare model, we use NASNet with 331×331 input, using pre-trained weight from Imagenet. Top layers are omitted, and replaced with a Dense layer of 1024 cells and 12 cells output layer for each class. Output activation is softmax, which is usual for multiclass classification.
#prepare model
img_width=331
img_height=331
network_notop = keras.applications.nasnet.NASNetLarge(input_shape=(img_width, img_height, 3),
include_top=False,
weights='imagenet', input_tensor=None,
pooling=None)
x = network_notop.output
x = GlobalAveragePooling2D()(x)
x = Dense(1024, activation='relu')(x)
x = BatchNormalization()(x)
predictions = Dense(12, activation='softmax')(x)
the_model = Model(network_notop.input, predictions)
Standard training.
Specific parameter for multiclass classification:
#training
learning_rate = 0.0001
logfile = session + '-train' + '.log'
batch_size=4
nbr_epochs=10
print("training directory: "+train_dir)
print("valication directory: "+valid_dir)
optimizer = SGD(lr=learning_rate, momentum=0.9, decay=0.0, nesterov=True)
the_model.compile(loss='categorical_crossentropy', optimizer=optimizer, metrics=['accuracy'])
csv_logger = CSVLogger(logfile, append=True)
early_stopping = EarlyStopping(monitor='val_loss', min_delta=0, patience=10, verbose=1, mode='auto')
best_model_filename=session+'-weights.{epoch:02d}-{val_loss:.2f}.h5'
best_model = ModelCheckpoint(best_model_filename, monitor='val_acc', verbose=1, save_best_only=True)
# this is the augmentation configuration we will use for training
train_datagen = ImageDataGenerator(
rescale=1. / 255,
shear_range=0.2,
zoom_range=0.2,
rotation_range=90,
width_shift_range=0.2,
height_shift_range=0.2,
horizontal_flip=True,
vertical_flip=True)
val_datagen = ImageDataGenerator(rescale=1. / 255)
print('prepare train generator')
train_generator = train_datagen.flow_from_directory(
train_dir,
target_size=(img_width, img_height),
batch_size=batch_size,
shuffle=True,
classes=classnames,
class_mode='categorical')
print('prepare validation generator')
validation_generator = val_datagen.flow_from_directory(
valid_dir,
target_size=(img_width, img_height),
batch_size=batch_size,
shuffle=True,
classes=classnames,
class_mode='categorical')
print('fit generator')
the_model.fit_generator(
generator=train_generator,
epochs=nbr_epochs,
verbose=1,
validation_data=validation_generator,
callbacks=[best_model, csv_logger, early_stopping])
Training progress
training directory: ../simpleNASNet/train
valication directory: ../simpleNASNet/valid
prepare train generator
Found 3800 images belonging to 12 classes.
prepare validation generator
Found 950 images belonging to 12 classes.
fit generator
Epoch 1/10
950/950 [==============================] - 635s 669ms/step - loss: 1.2295 - acc: 0.6039 - val_loss: 0.6469 - val_acc: 0.7979
Epoch 00001: val_acc improved from -inf to 0.79789, saving model to simpleNASNet-weights.01-0.65.h5
Epoch 2/10
950/950 [==============================] - 557s 586ms/step - loss: 0.6281 - acc: 0.7929 - val_loss: 0.3840 - val_acc: 0.8674
Epoch 00002: val_acc improved from 0.79789 to 0.86737, saving model to simpleNASNet-weights.02-0.38.h5
Epoch 3/10
950/950 [==============================] - 557s 586ms/step - loss: 0.5220 - acc: 0.8345 - val_loss: 0.3026 - val_acc: 0.9000
Epoch 00003: val_acc improved from 0.86737 to 0.90000, saving model to simpleNASNet-weights.03-0.30.h5
Epoch 4/10
950/950 [==============================] - 558s 587ms/step - loss: 0.4369 - acc: 0.8566 - val_loss: 0.2830 - val_acc: 0.9105
Epoch 00004: val_acc improved from 0.90000 to 0.91053, saving model to simpleNASNet-weights.04-0.28.h5
Epoch 5/10
950/950 [==============================] - 558s 588ms/step - loss: 0.3722 - acc: 0.8842 - val_loss: 0.2310 - val_acc: 0.9253
Epoch 00005: val_acc improved from 0.91053 to 0.92526, saving model to simpleNASNet-weights.05-0.23.h5
Epoch 6/10
950/950 [==============================] - 559s 588ms/step - loss: 0.3213 - acc: 0.8966 - val_loss: 0.2210 - val_acc: 0.9232
Epoch 00006: val_acc did not improve from 0.92526
Epoch 7/10
950/950 [==============================] - 556s 585ms/step - loss: 0.3202 - acc: 0.8939 - val_loss: 0.2190 - val_acc: 0.9263
Epoch 00007: val_acc improved from 0.92526 to 0.92632, saving model to simpleNASNet-weights.07-0.22.h5
Epoch 8/10
950/950 [==============================] - 559s 589ms/step - loss: 0.2997 - acc: 0.9063 - val_loss: 0.1861 - val_acc: 0.9389
Epoch 00008: val_acc improved from 0.92632 to 0.93895, saving model to simpleNASNet-weights.08-0.19.h5
Epoch 9/10
950/950 [==============================] - 554s 584ms/step - loss: 0.2469 - acc: 0.9203 - val_loss: 0.1942 - val_acc: 0.9379
Epoch 00009: val_acc did not improve from 0.93895
Epoch 10/10
950/950 [==============================] - 557s 587ms/step - loss: 0.2619 - acc: 0.9147 - val_loss: 0.1695 - val_acc: 0.9421
Epoch 00010: val_acc improved from 0.93895 to 0.94211, saving model to simpleNASNet-weights.10-0.17.h5
Caution: test files must be put into a directory under /data/test. For simplicity, we create /data/test/0, but any directory name is okay, as long as it is under /data/test . This behavior is quite strange, but maybe to make flow_from_directory() work the same way for training phase and prediction/inference phase.
#prediction
batch_size=4
nbr_test_samples=794
img_width=331
img_height=331
#choose weights file manually
weights_path = 'simpleNASNet-weights.10-0.17.h5' # choose file manually, filename may be different
test_data_dir = '../data/test/'
test_datagen = ImageDataGenerator(rescale=1./255)
test_generator = test_datagen.flow_from_directory(
test_data_dir,
target_size=(img_width, img_height),
batch_size=batch_size,
shuffle = False, # no shuffling, since filenames must match predictions. Shuffling may change file sequence
classes = None, #
class_mode = None)
test_image_list = test_generator.filenames
print('Loading model and weights')
predict_model = load_model(weights_path)
print('Begin to predict for testing data ...')
predictions = predict_model.predict_generator(test_generator, nbr_test_samples)
np.savetxt(session+'-predictions.txt', predictions) # store prediction matrix, for later analysis if necessary
Constructing submission file
#submission
submission_file=session+'-submit.csv'
print('Begin to write submission file:'+submission_file)
f_submit = open(submission_file, 'w')
f_submit.write('file,speciesn')
for i, image_name in enumerate(test_image_list):
# find maximum prediction of 12
max_index=0
max_value=0
for x in range(0, 12):
if(predictions[i][x]>max_value):
max_value=predictions[i][x]
max_index=x
basename=os.path.basename(image_name)
prediction_class = classnames[max_index] # get predictions from array
f_submit.write('%s,%sn' % (basename, prediction_class))
f_submit.close()
print('Finished write submission file ..')
To check final score, let’s go to Late Submission page in Kaggle Plant Seedlings Classification. The score is 0.96095, which ranks about 400 in leaderboard.
![]() |
Late submission score |
This article is a simple introduction to simple binary classification for images with Keras deep learning library.
There are many ways to do image classification with Keras. Here are the detail of this particular implementation:
![]() |
Dogs vs Cats classification problem |
First step is to prepare working directory.
![]() |
||
Binary classification directory structure |
This is the directory structure used in this article.
It’s better to use a structured working directory, don’t just mix all files in the same directory. You may modify the directory structure to suit your needs.
flow_from_director() expects each class to have its own directory. The directory names must match class names.
Now we can jump straight into the code. First step is to import libraries.
import tensorflow as tf
import keras as keras
import os
from keras.layers import Flatten, Dense, AveragePooling2D, GlobalAveragePooling2D
from keras.models import Model
from keras.optimizers import RMSprop, SGD
from keras.callbacks import ModelCheckpoint
from keras.callbacks import EarlyStopping
from keras.preprocessing.image import ImageDataGenerator
from keras.callbacks import CSVLogger
from keras.layers.normalization import BatchNormalization
import numpy as np
from keras.models import load_model
import numpy as np
from pathlib import Path
import os
import shutil
The next step is to define parameters for our deep learning model.
# preparing parameters
image_dir_cat='../data/train/cat' # assuming cat & dog images has been separated in different directories
image_dir_dog='../data/train/dog'
session = "simple1000" # to differentiate between runs
ClassNames = ['cat', 'dog']
data_dir="../simple1000" # to differentiate between runs
learning_rate = 0.0001
img_width = 331 # 331 for pre-trained nasnet
img_height = 331
nbr_epochs = 10
batch_size = 4 # batch size depends on available memory on GPU. GTX 1080 Ti use (4)
np.random.seed(2018)
train_dir = data_dir + "/train"
valid_dir = data_dir + "/valid"
number_of_class=len(ClassNames)
print("train directory : ", train_dir)
print("valid directory : ", valid_dir)
print("number of classes: "+ str(number_of_class))
logfile = session + '-train' + '.log'
print("logfile :", logfile)
Explanation:
The next step is to prepare files for training step. We have 12500 images of cats and 12500 images of dogs in the dataset, but in this experiment, we only use 1000 images of cats and 1000 of dogs , to speed up the experiment. We can easily add more files later.
The following code prepares files for the training. For training we use 800 cat images and 800 dog images, while for validation we use 200 cat images and 200 dog images.
# make training directory
# make validation directory
# copy images to respective directories
print("copy start")
def MakeDir(newdir):
if not os.path.exists(newdir):
os.makedirs(newdir)
# make validation & training directories, if not exist yet
MakeDir(valid_dir)
MakeDir(valid_dir+'/cat')
MakeDir(valid_dir+'/dog')
MakeDir(train_dir)
MakeDir(train_dir+'/cat')
MakeDir(train_dir+'/dog')
# copy files to working directories
print("copy cats")
counter=0
for root, dirs, files in os.walk(image_dir_cat):
for file in files:
fullfilename = os.path.join(root, file)
# print(str(counter) + ": " + fullfilename)
if(counter<800):
shutil.copyfile(fullfilename,train_dir+"/cat/"+file)
if(counter>=800 and counter<1000):
shutil.copyfile(fullfilename,valid_dir+"/cat/"+file)
if(counter>=1000):
break
counter=counter+1
print("copy dogs")
counter=0
for root, dirs, files in os.walk(image_dir_dog):
for file in files:
fullfilename = os.path.join(root, file)
# print(str(counter) + ": " + fullfilename)
if(counter<800):
shutil.copyfile(fullfilename,train_dir+"/dog/"+file)
if(counter>=800 and counter<1000):
shutil.copyfile(fullfilename,valid_dir+"/dog/"+file)
if(counter>=1000):
break
counter=counter+1
print("copy finished")
# make model with transfer learning
if(True):
model_notop = keras.applications.nasnet.NASNetLarge(input_shape=(img_width, img_height, 3),
include_top=False,
weights='imagenet', input_tensor=None,
pooling=None)
# add a global spatial average pooling layer
x = model_notop.output
x = GlobalAveragePooling2D()(x)
x = Dense(1024, activation='relu')(x) # let's add a fully-connected layer
x = BatchNormalization()(x)
predictions = Dense(1, activation='sigmoid')(x)
deep_model = Model(model_notop.input, predictions)
Explanation
# training
if(True):
sgd_optimizer = SGD(lr=learning_rate, momentum=0.9, decay=0.0, nesterov=True)
deep_model.compile(loss='binary_crossentropy', optimizer=sgd_optimizer, metrics=['accuracy'])
# set up callbacks
csv_logger = CSVLogger(logfile, append=True)
early_stopping = EarlyStopping(monitor='val_loss', min_delta=0, patience=2, verbose=1, mode='auto')
best_model_file=session+'-weights.{epoch:02d}-{val_loss:.2f}.h5'
# best_model_file = session + '-weights' + '.h5'
best_model = ModelCheckpoint(best_model_file, monitor='val_acc', verbose=1, save_best_only=True)
# this is the augmentation configuration we will use for training
train_datagen = ImageDataGenerator(
rescale=1. / 255,
shear_range=0.2,
zoom_range=0.2,
rotation_range=90,
width_shift_range=0.2,
height_shift_range=0.2,
horizontal_flip=True,
vertical_flip=True)
val_datagen = ImageDataGenerator(rescale=1. / 255)
print('prepare train generator')
train_generator = train_datagen.flow_from_directory(
train_dir,
target_size=(img_width, img_height),
batch_size=batch_size,
shuffle=True,
class_mode='binary')
print('prepare validation generator')
validation_generator = val_datagen.flow_from_directory(
valid_dir,
target_size=(img_width, img_height),
batch_size=batch_size,
shuffle=True,
class_mode='binary')
print('fit generator')
deep_model.fit_generator(
generator=train_generator,
# steps_per_epoch=nbr_train_samples/batch_size, # in Keras 2.2.0, automatically acquired from train generator
epochs=nbr_epochs,
verbose=1,
validation_data=validation_generator,
# validation_steps=nbr_validation_samples/batch_size, # automatically acquired from validation generator
callbacks=[best_model, csv_logger, early_stopping])
training progress
prepare train generator
Found 1600 images belonging to 2 classes.
prepare validation generator
Found 400 images belonging to 2 classes.
fit generator
Epoch 1/10
400/400 [==============================] - 279s 697ms/step - loss: 0.3509 - acc: 0.8500 - val_loss: 0.1920 - val_acc: 0.9525
Epoch 00001: val_acc improved from -inf to 0.95250, saving model to simple1000-weights.01-0.19.h5
Epoch 2/10
400/400 [==============================] - 230s 574ms/step - loss: 0.3015 - acc: 0.8769 - val_loss: 0.1307 - val_acc: 0.9725
Epoch 00002: val_acc improved from 0.95250 to 0.97250, saving model to simple1000-weights.02-0.13.h5
Epoch 3/10
400/400 [==============================] - 231s 578ms/step - loss: 0.2886 - acc: 0.8869 - val_loss: 0.1337 - val_acc: 0.9675
Epoch 00003: val_acc did not improve from 0.97250
Epoch 4/10
400/400 [==============================] - 233s 581ms/step - loss: 0.3108 - acc: 0.8744 - val_loss: 0.1299 - val_acc: 0.9750
Epoch 00004: val_acc improved from 0.97250 to 0.97500, saving model to simple1000-weights.04-0.13.h5
Epoch 5/10
400/400 [==============================] - 232s 580ms/step - loss: 0.2880 - acc: 0.8863 - val_loss: 0.1093 - val_acc: 0.9775
Epoch 00005: val_acc improved from 0.97500 to 0.97750, saving model to simple1000-weights.05-0.11.h5
Epoch 6/10
400/400 [==============================] - 231s 576ms/step - loss: 0.2284 - acc: 0.9113 - val_loss: 0.0928 - val_acc: 0.9775
Epoch 00006: val_acc did not improve from 0.97750
Epoch 7/10
400/400 [==============================] - 230s 575ms/step - loss: 0.2560 - acc: 0.8969 - val_loss: 0.0935 - val_acc: 0.9825
Epoch 00007: val_acc improved from 0.97750 to 0.98250, saving model to simple1000-weights.07-0.09.h5
Epoch 8/10
400/400 [==============================] - 231s 577ms/step - loss: 0.2461 - acc: 0.9019 - val_loss: 0.0821 - val_acc: 0.9775
Epoch 00008: val_acc did not improve from 0.98250
Epoch 9/10
400/400 [==============================] - 231s 578ms/step - loss: 0.2606 - acc: 0.8981 - val_loss: 0.0722 - val_acc: 0.9825
Epoch 00009: val_acc did not improve from 0.98250
Epoch 10/10
400/400 [==============================] - 231s 578ms/step - loss: 0.2267 - acc: 0.9113 - val_loss: 0.1130 - val_acc: 0.9775
Epoch 00010: val_acc did not improve from 0.98250
Prediction step
#prediction
nbr_test_samples=12500
#choose weights file manually
weights_path = 'simple1000-weights.07-0.09.h5'
test_data_dir = '../data/test/'
test_datagen = ImageDataGenerator(rescale=1./255)
test_generator = test_datagen.flow_from_directory(
test_data_dir,
target_size=(img_width, img_height),
batch_size=batch_size,
shuffle = False, # no shuffling, since filenames must match predictions. Shuffling may change file sequence
classes = None, #
class_mode = None)
test_image_list = test_generator.filenames
print('Loading model and weights')
predict_model = load_model(weights_path)
print('Begin to predict for testing data ...')
predictions = predict_model.predict_generator(test_generator, nbr_test_samples)
np.savetxt(session+'-predictions.txt', predictions) # store prediction matrix, for later analysis if necessary
Make submission file, format must match given sample_submission.csv
# submission
submission_file=session+'-submit.csv'
print('Begin to write submission file:'+submission_file)
f_submit = open(submission_file, 'w')
f_submit.write('id,labeln')
for i, image_name in enumerate(test_image_list):
basename=os.path.basename(image_name)
filename, fileext = os.path.splitext(basename)
prediction_class =predictions[i][0] # get predictions from array
f_submit.write('%s,%sn' % (filename, prediction_class))
f_submit.close()
print('Finished write submission file ..')
Submit the result to https://www.kaggle.com/c/dogs-vs-cats-redux-kernels-edition/leaderboard , click on “Late Submission”
We got score of 0.10979, still long way from the top (0.03) but not too bad for only 1000 samples.
Full source code for simple solution is available here: https://github.com/waskita/kaggle-dogs-cats/blob/master/simple-binary-classification.ipynb
Download the Developer’s Guide to Building AI Applications:
https://info.microsoft.com/ww-landing-ai-developers-bot-ebook.html
Create your first intelligent bot with Microsoft AI.
Artificial intelligence (AI) is accelerating the digital transformation for every industry, with examples spanning manufacturing, retail, finance, healthcare, and many others. At this rate, every industry will be able to use AI to amplify human ingenuity. In this e-book, Anand Raman and Wee Hyong Tok from Microsoft provide a comprehensive roadmap for developers to build their first AI-infused application.
Using a Conference Buddy as an example, you’ll learn the key ingredients needed to develop an intelligent chatbot that helps conference participants interact with speakers. This e-book provides a gentle introduction to the tools, infrastructure, and services on the Microsoft AI Platform, and teaches you how to create powerful, intelligent applications.