https://pubs.acs.org/doi/10.1021/acsnano.3c01544
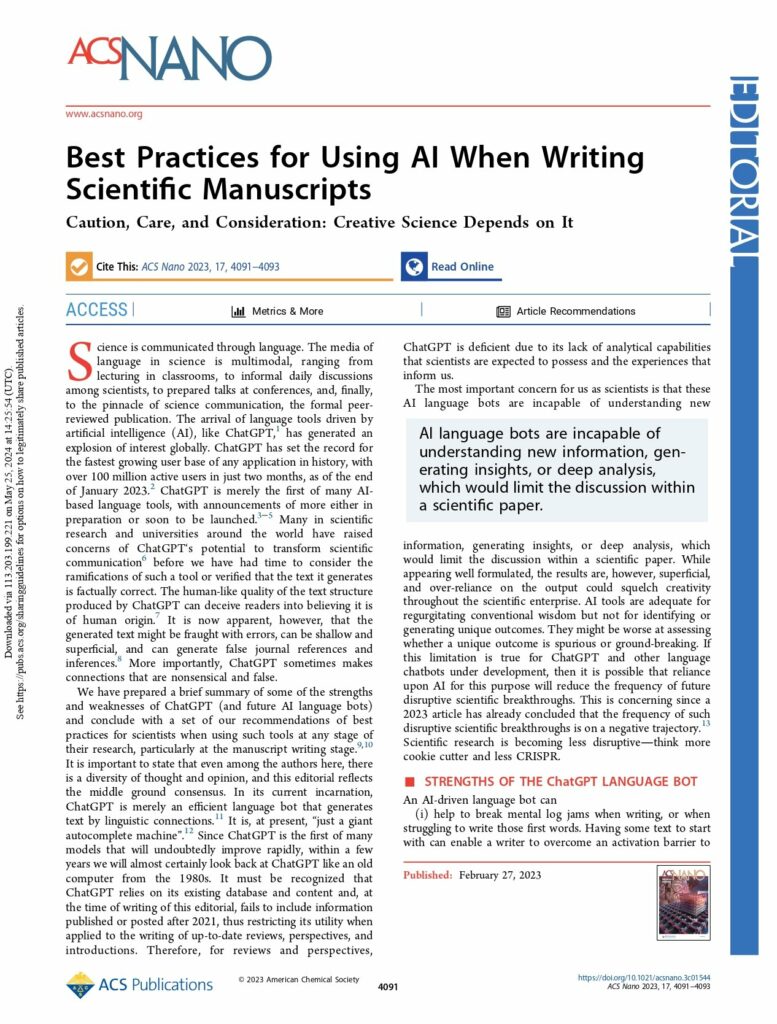
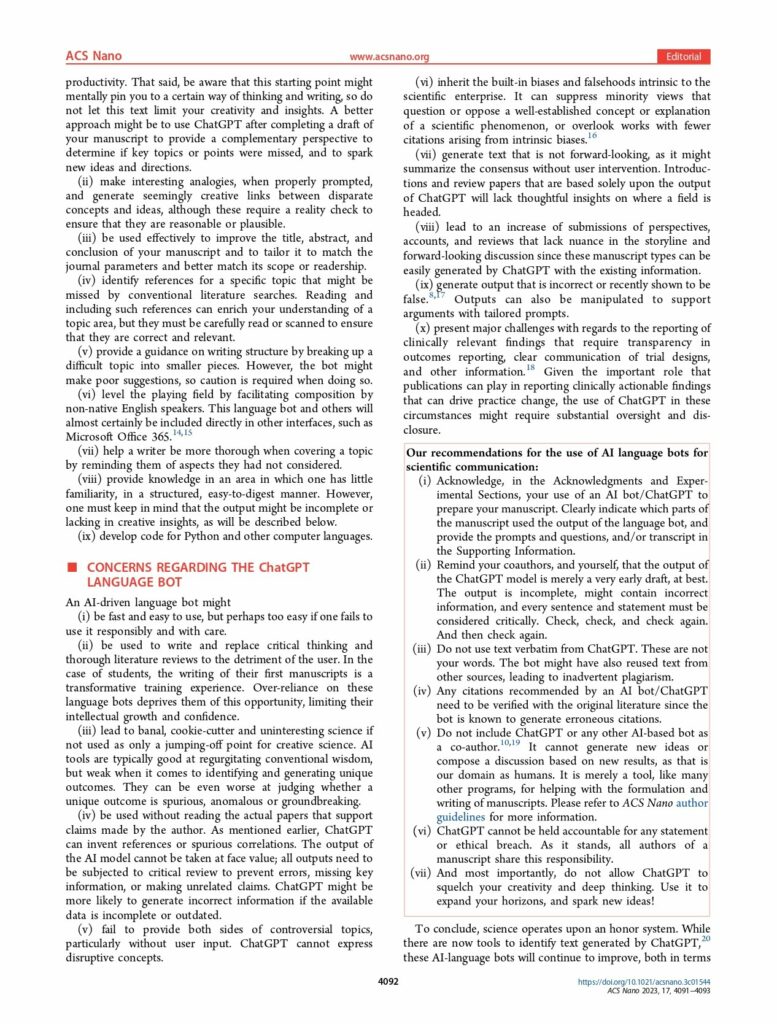
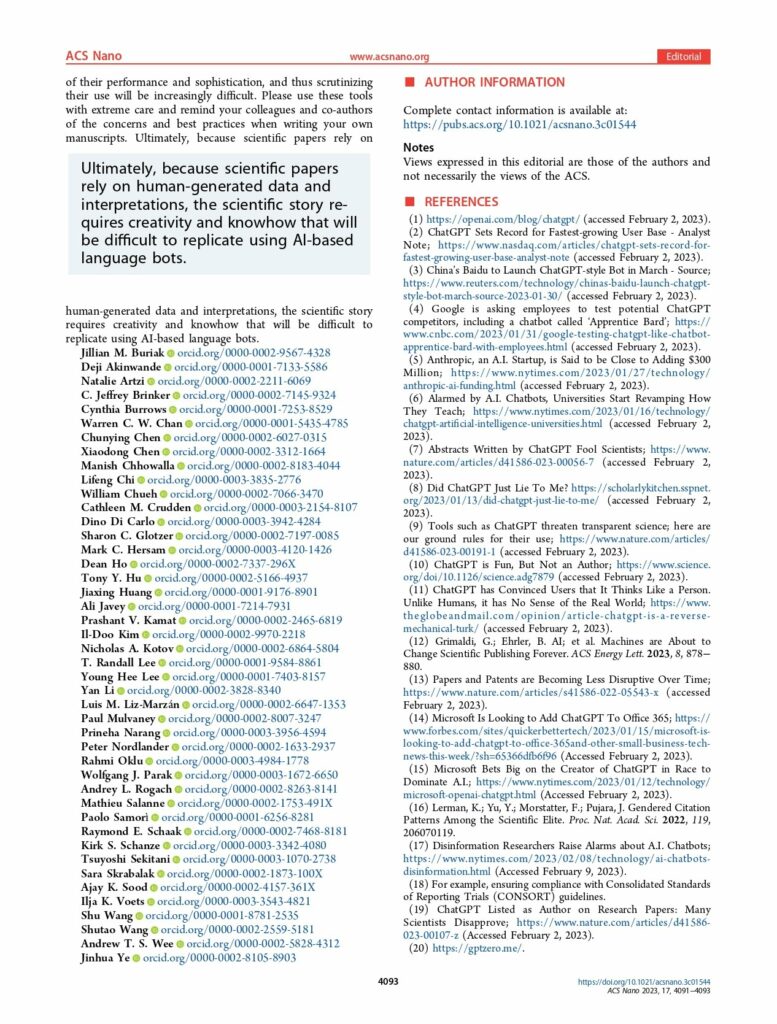
Artikel Saintek Umum
The Computer Language Benchmark Game, also known as “The Benchmarks Game” or previously as “The Great Computer Language Shootout,” is a project that compares the performance of different programming languages using a set of benchmark problems. It’s a valuable resource for researchers comparing language performance.
Here are a few papers from recent years that have utilized CLBG:
These papers cover various aspects of the topic, including:
Systematic Review | Literature Review | |
---|---|---|
Definition | High-level overview of primary research on a focused question that identifies, selects, synthesizes, and appraises all high quality research evidence relevant to that question | Qualitatively summarizes evidence on a topic using informal or subjective methods to collect and interpret studies |
Goals | Answers a focused clinical question Eliminate bias |
Provide summary or overview of topic |
Question | Clearly defined and answerable clinical question Recommend using PICO as a guide |
Can be a general topic or a specific question |
Components | Pre-specified eligibility criteria Systematic search strategy Assessment of the validity of findings Interpretation and presentation of results Reference list |
Introduction Methods Discussion Conclusion Reference list |
Number of Authors | Three or more | One or more |
Timeline | Months to years Average eighteen months |
Weeks to months |
Requirement | Thorough knowledge of topic Perform searches of all relevant databases Statistical analysis resources (for meta-analysis) |
Understanding of topic |
Value | Connects practicing clinicians to high quality evidence Supports evidence-based practice |
Provides summary of literature on the topic |
Source: Lynn Kysh, What’s in a name? The difference between a Systematic Review and a Literature Review, and why it matters
For ICL, it discusses key design decisions like exemplar quantity, ordering, label quality, format, and similarity that critically influence output quality. It also covers ICL techniques like K-Nearest Neighbor exemplar selection.
Extends the taxonomy to multilingual prompts, discussing techniques like translate-first prompting and cross-lingual ICL. It also covers multimodal prompts spanning image, audio, video, segmentation, and 3D modalities.
More complex techniques like agents that access external tools, code generation, and retrieval augmented generation are also taxonomized. Evaluation techniques using LLMs are discussed.
Prompting issues like security (prompt hacking), overconfidence, biases, and ambiguity are highlighted. Two case studies – benchmarking techniques on MMLU and an entrapment detection prompt engineering exercise – are presented.
Problem:
undefined reference to `boost::this_thread::disable_interruption::~disable_interruption()’
Solution:
Add options to g++ / clang++
-lboost_thread -lboost_system
Reference
Here are some papers that explore Rust’s energy efficiency:
1. “Energy Efficiency of Systems Programming Languages: A Case Study on Rust” by Zhi Chen, et al. (2020)
This paper compares the energy efficiency of several programming languages, including C, C++, Java, and Rust. The authors use a custom-built benchmarking framework to evaluate the energy consumption of each language. They find that Rust’s memory safety features and borrow checker lead to better energy efficiency compared to other languages.
Paper link: [PDF](https://www.cs.cornell.edu/~yizhang/papers/chen-2019-energy-efficiency.pdf)
2. “Rust, a Language for System Programming with Energy Efficiency in Mind” by Niklaus Wirth (2018)
In this paper, the author discusses Rust’s design principles and how they contribute to energy efficiency. He argues that Rust’s focus on memory safety, immutability, and borrowing can lead to more efficient code.
Paper link: [PDF](https://www.inf.ethz.ch/personal/niklauswirth/2018-rust-energy-efficiency.pdf)
3. “Energy Efficiency of Memory Management in Rust” by Yizhang Zhang, et al. (2020)
This paper investigates the energy efficiency of Rust’s memory management system, which is based on ownership and borrowing. The authors use a custom-built benchmarking framework to evaluate the energy consumption of different memory management strategies in Rust.
Paper link: [PDF](https://www.cs.cornell.edu/~yizhang/papers/zhang-2020-energy-efficiency.pdf)
4. “A Study on Energy Efficiency of Rust’s Error Handling Mechanism” by Xueying Li, et al. (2020)
In this paper, the authors analyze the energy efficiency of Rust’s error handling mechanism, which is designed to reduce the overhead of error propagation and handling. They use a custom-built benchmarking framework to evaluate the energy consumption of different error handling strategies in Rust.
Paper link: [PDF](https://www.researchgate.net/publication/339311345_A_Study_on_Energy_Efficiency_of_Rust’s_Error_Handling_Mechanism)
Original paper: “Energy efficiency across programming languages: how do energy, time, and memory relate?“
Original link: https://sites.google.com/view/energy-efficiency-languages/results?authuser=0
binary-trees
fannkuch-redux
fasta
k-nucleotide
mandelbrot
n-body
pidigits
regex-redux
reverse-complement
spectral-norm
D. Pareto Optimal Set
You can now train a 70b language model at home https://www.answer.ai/posts/2024-03-06-fsdp-qlora.html