URL: https://zindi.africa/competitions/ai4d-african-language-dataset-challenge
In recent times, pre-trained language models have led to significant improvement in various Natural Language Processing (NLP) tasks and transfer learning is rapidly changing the field. Transfer Learning is the process of training a model on a large-scale dataset and then using that pre-trained model to conduct learning for another downstream task (i.e. a target task like name entity recognition).
Among leading architectures for pre-training models for transfer learning in NLP, pre-trained models in African languages are barely represented mainly due to a lack of data. (However, there are some examples, for example this multilingual BERT that includes likes like Swahili and Yoruba.) While these architectures are freely available for use, most are data-hungry. The GPT-2 model, for instance, used millions, possibly billions of text to train. (ref)
This gap exists due to a lack of availability of data for African languages on the Internet. The languages selected for BERT pre-training “were chosen because they are the top languages with the largest Wikipedias”. (ref) Similarly, the 157 pre-trained language models made available by fastText were trained on Wikipedia and Common Crawl. (ref)
Therefore, this challenge’s objective is the creation, curation and collation of good quality African language datasets for a specific NLP task. This task-specific NLP dataset will serve as the downstream task we can evaluate future language models on.
This challenge is sponsored by GIZ and is hosted in partnership with the Artificial Intelligence for Development Africa(AI4D-Africa) Network.
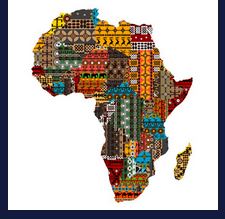