URL: https://openai.com/blog/procgen-minerl-competitions/
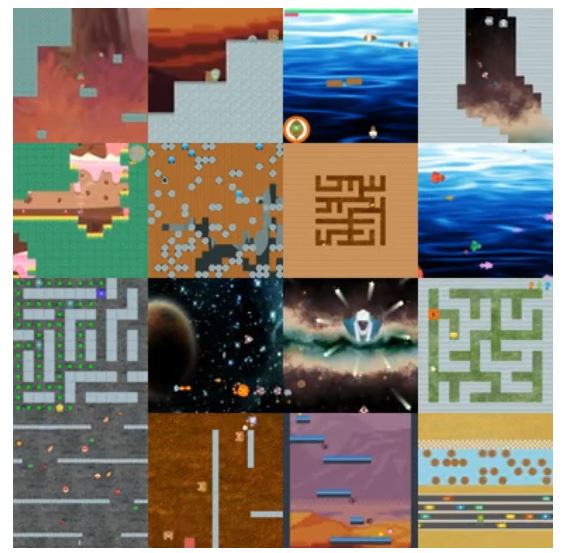
AIcrowd challenge: https://www.aicrowd.com/challenges/aicrowd-blitz-may-2020
Team SimcovID sedang membahas model dan simulasi tentang COVID-19 di Indonesia
Update terakhir dapat dilihat di https://simcovid.github.io/
Update 20 April 2020: https://simcovid.github.io/topics/simulation_model/modelling/report/Modelling%20Update%20SimcovID%20220420.pdf
Link: https://www.aicrowd.com/challenges/food-recognition-challenge
Recognizing food from images is an extremely useful tool for a variety of use cases. In particular, it would allow people to track their food intake by simply taking a picture of what they consume. Food tracking can be of personal interest, and can often be of medical relevance as well. Medical studies have for some time been interested in the food intake of study participants, but had to rely on food frequency questionnaires that are known to be imprecise.
Image-based food recognition has in the past few years made substantial progress thanks to advances in deep learning. But food recognition remains a difficult problem for a variety of reasons.
The goal of this challenge is to train models which can look at images of food items and detect the individual food items present in them. We use a novel dataset of food images collected through the MyFoodRepo app where numerous volunteer Swiss users provide images of their daily food intake in the context of a digital cohort called Food & You. This growing data set has been annotated – or automatic annotations have been verified – with respect to segmentation, classification (mapping the individual food items onto an ontology of Swiss Food items), and weight / volume estimation.
This is an evolving dataset, where we will release more data as the dataset grows over time.
URL: http://www.mlebook.com/wiki/doku.php
DeepDR Diabetic Retinopathy Image Dataset (DeepDRiD) Challenge.
The aim of this challenge is to evaluate algorithms for automated fundus image quality estimation and grading of diabetic retinopathy.
Diabetic Retinopathy (DR) is the most prevalent cause of avoidable vision impairment, mainly affecting the working-age population in the world. Early diagnosis and timely treatment of diabetic retinopathy help in preventing blindness. This objective can be accomplished by a coordinated effort to organize large, regular screening programs. Particularly computer-assisted ones. Well-acquired, large, and diverse retinal image datasets are essential for developing and testing digital screening programs and the automated algorithms at their core. Therefore, we provide a large retinal image dataset, DeepDR (Deep Diabetic Retinopathy), to facilitate the following investigations in the community. First, unlike previous studies, in order to further promote the early diagnosis precision and robustness in practice, we provide the dual-view fundus images from the same eyes, e.g. the optic disc as the center and the fovea as the center, to classify and grade DR lesions. The expected results should outperform the state-of-the-art models built with the single-view fundus images. Second, we include various image quality of fundus images in DeepDR dataset to reflect the real scenario in practice. We expect to build a model that can estimate the image quality level to provide supportive guidance to the fundus image-based diagnosis. Lastly, to explore the extreme generalizability of a DR grading system, we desire to build a model that transfer the capability of DR diagnosis learned from a large number of regular fundus images to the ultra-widefield retinal images. Usually, we use the regular fundus images for initial screening; the widefield scanning performs as a further screening mean because it can provide complete eye information. To the best of our knowledge, our database, DeepDR (Deep Diabetic Retinopathy), is the largest database of DR patient population, and provide more than 1,000 patients data. In addition, it is the only dataset constituting dual-view fundus images from the same eyes and various distinguishable quality levels images. This data set provides information on the disease severity of diabetic retinopathy, and image quality level for each image.
Moreover, we provide the first ultra-widefield retinal image dataset to facilitate the study of model generalizability and meanwhile further extend the DR diagnose means from traditional fundus imaging to wide-field retinal photography. This makes it perfect for development and evaluation of image analysis algorithms for early detection of diabetic retinopathy.
The challenge is subdivided into three tasks as follows (participants can submit results for at least one of the challenges):
● Disease Grading: Classification of fundus images according to the severity level of diabetic retinopathy using dual view retinal fundus images. For more details please refer to Sub-challenge 1.
● Image Quality Estimation: Fundus quality assessment for overall image quality, artifacts, clarity, and field definition. For more details please refer to Sub-challenge 2.
● Transfer Learning: Explore the generalizability of a Diabetic Retinopathy (DR) grading system. For more details please refer to Sub-challenge 3.