This article is a simple introduction to simple binary classification for images with Keras deep learning library.
There are many ways to do image classification with Keras. Here are the detail of this particular implementation:
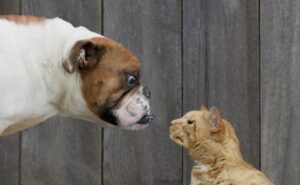 |
Dogs vs Cats classification problem |
Prepare Working Directories
First step is to prepare working directory.
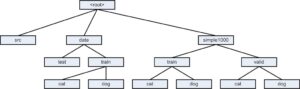 |
Binary classification directory structure |
|
|
This is the directory structure used in this article.
It’s better to use a structured working directory, don’t just mix all files in the same directory. You may modify the directory structure to suit your needs.
flow_from_director() expects each class to have its own directory. The directory names must match class names.
Download Dataset
- Download dataset from Dataset: Dogs vs Cats Redux: Kernels Edition
- Put cat images in <root>/data/train/cat
- Put dog images in <root>/data/train/dog
- Put test images in <root>/data/test
Now we can jump straight into the code. First step is to import libraries.
import tensorflow as tf
import keras as keras
import os
from keras.layers import Flatten, Dense, AveragePooling2D, GlobalAveragePooling2D
from keras.models import Model
from keras.optimizers import RMSprop, SGD
from keras.callbacks import ModelCheckpoint
from keras.callbacks import EarlyStopping
from keras.preprocessing.image import ImageDataGenerator
from keras.callbacks import CSVLogger
from keras.layers.normalization import BatchNormalization
import numpy as np
from keras.models import load_model
import numpy as np
from pathlib import Path
import os
import shutil
The next step is to define parameters for our deep learning model.
# preparing parameters
image_dir_cat='../data/train/cat' # assuming cat & dog images has been separated in different directories
image_dir_dog='../data/train/dog'
session = "simple1000" # to differentiate between runs
ClassNames = ['cat', 'dog']
data_dir="../simple1000" # to differentiate between runs
learning_rate = 0.0001
img_width = 331 # 331 for pre-trained nasnet
img_height = 331
nbr_epochs = 10
batch_size = 4 # batch size depends on available memory on GPU. GTX 1080 Ti use (4)
np.random.seed(2018)
train_dir = data_dir + "/train"
valid_dir = data_dir + "/valid"
number_of_class=len(ClassNames)
print("train directory : ", train_dir)
print("valid directory : ", valid_dir)
print("number of classes: "+ str(number_of_class))
logfile = session + '-train' + '.log'
print("logfile :", logfile)
Explanation:
- image_dir_cat & image_dir_dog must match the directory where we put our training dataset.
- session string is useful if we want to make several different run. There will be many weights files, prediction files. If we don’t stick to a naming structure, the whole thing can become a jumbled mess
The next step is to prepare files for training step. We have 12500 images of cats and 12500 images of dogs in the dataset, but in this experiment, we only use 1000 images of cats and 1000 of dogs , to speed up the experiment. We can easily add more files later.
The following code prepares files for the training. For training we use 800 cat images and 800 dog images, while for validation we use 200 cat images and 200 dog images.
# make training directory
# make validation directory
# copy images to respective directories
print("copy start")
def MakeDir(newdir):
if not os.path.exists(newdir):
os.makedirs(newdir)
# make validation & training directories, if not exist yet
MakeDir(valid_dir)
MakeDir(valid_dir+'/cat')
MakeDir(valid_dir+'/dog')
MakeDir(train_dir)
MakeDir(train_dir+'/cat')
MakeDir(train_dir+'/dog')
# copy files to working directories
print("copy cats")
counter=0
for root, dirs, files in os.walk(image_dir_cat):
for file in files:
fullfilename = os.path.join(root, file)
# print(str(counter) + ": " + fullfilename)
if(counter<800):
shutil.copyfile(fullfilename,train_dir+"/cat/"+file)
if(counter>=800 and counter<1000):
shutil.copyfile(fullfilename,valid_dir+"/cat/"+file)
if(counter>=1000):
break
counter=counter+1
print("copy dogs")
counter=0
for root, dirs, files in os.walk(image_dir_dog):
for file in files:
fullfilename = os.path.join(root, file)
# print(str(counter) + ": " + fullfilename)
if(counter<800):
shutil.copyfile(fullfilename,train_dir+"/dog/"+file)
if(counter>=800 and counter<1000):
shutil.copyfile(fullfilename,valid_dir+"/dog/"+file)
if(counter>=1000):
break
counter=counter+1
print("copy finished")
Building Model
# make model with transfer learning
if(True):
model_notop = keras.applications.nasnet.NASNetLarge(input_shape=(img_width, img_height, 3),
include_top=False,
weights='imagenet', input_tensor=None,
pooling=None)
# add a global spatial average pooling layer
x = model_notop.output
x = GlobalAveragePooling2D()(x)
x = Dense(1024, activation='relu')(x) # let's add a fully-connected layer
x = BatchNormalization()(x)
predictions = Dense(1, activation='sigmoid')(x)
deep_model = Model(model_notop.input, predictions)
Explanation
- For the first layers, we use model & weight from NASNet, without its fully connected layer.
- We replace the NASNet final layer with our own, with 1024 hidden neurons (Dense) and 1 in output layer.
- Since this is a binary classification, the final layer activation is sigmoid, and only consist of 1 cell.
- Batch Normalization is added to reduce overfitting
- The number of hidden layer (1024) is arbitrary, it can be increased or decreased later to find better result.
Train The Model
# training
if(True):
sgd_optimizer = SGD(lr=learning_rate, momentum=0.9, decay=0.0, nesterov=True)
deep_model.compile(loss='binary_crossentropy', optimizer=sgd_optimizer, metrics=['accuracy'])
# set up callbacks
csv_logger = CSVLogger(logfile, append=True)
early_stopping = EarlyStopping(monitor='val_loss', min_delta=0, patience=2, verbose=1, mode='auto')
best_model_file=session+'-weights.{epoch:02d}-{val_loss:.2f}.h5'
# best_model_file = session + '-weights' + '.h5'
best_model = ModelCheckpoint(best_model_file, monitor='val_acc', verbose=1, save_best_only=True)
# this is the augmentation configuration we will use for training
train_datagen = ImageDataGenerator(
rescale=1. / 255,
shear_range=0.2,
zoom_range=0.2,
rotation_range=90,
width_shift_range=0.2,
height_shift_range=0.2,
horizontal_flip=True,
vertical_flip=True)
val_datagen = ImageDataGenerator(rescale=1. / 255)
print('prepare train generator')
train_generator = train_datagen.flow_from_directory(
train_dir,
target_size=(img_width, img_height),
batch_size=batch_size,
shuffle=True,
class_mode='binary')
print('prepare validation generator')
validation_generator = val_datagen.flow_from_directory(
valid_dir,
target_size=(img_width, img_height),
batch_size=batch_size,
shuffle=True,
class_mode='binary')
print('fit generator')
deep_model.fit_generator(
generator=train_generator,
# steps_per_epoch=nbr_train_samples/batch_size, # in Keras 2.2.0, automatically acquired from train generator
epochs=nbr_epochs,
verbose=1,
validation_data=validation_generator,
# validation_steps=nbr_validation_samples/batch_size, # automatically acquired from validation generator
callbacks=[best_model, csv_logger, early_stopping])
training progress
prepare train generator
Found 1600 images belonging to 2 classes.
prepare validation generator
Found 400 images belonging to 2 classes.
fit generator
Epoch 1/10
400/400 [==============================] - 279s 697ms/step - loss: 0.3509 - acc: 0.8500 - val_loss: 0.1920 - val_acc: 0.9525
Epoch 00001: val_acc improved from -inf to 0.95250, saving model to simple1000-weights.01-0.19.h5
Epoch 2/10
400/400 [==============================] - 230s 574ms/step - loss: 0.3015 - acc: 0.8769 - val_loss: 0.1307 - val_acc: 0.9725
Epoch 00002: val_acc improved from 0.95250 to 0.97250, saving model to simple1000-weights.02-0.13.h5
Epoch 3/10
400/400 [==============================] - 231s 578ms/step - loss: 0.2886 - acc: 0.8869 - val_loss: 0.1337 - val_acc: 0.9675
Epoch 00003: val_acc did not improve from 0.97250
Epoch 4/10
400/400 [==============================] - 233s 581ms/step - loss: 0.3108 - acc: 0.8744 - val_loss: 0.1299 - val_acc: 0.9750
Epoch 00004: val_acc improved from 0.97250 to 0.97500, saving model to simple1000-weights.04-0.13.h5
Epoch 5/10
400/400 [==============================] - 232s 580ms/step - loss: 0.2880 - acc: 0.8863 - val_loss: 0.1093 - val_acc: 0.9775
Epoch 00005: val_acc improved from 0.97500 to 0.97750, saving model to simple1000-weights.05-0.11.h5
Epoch 6/10
400/400 [==============================] - 231s 576ms/step - loss: 0.2284 - acc: 0.9113 - val_loss: 0.0928 - val_acc: 0.9775
Epoch 00006: val_acc did not improve from 0.97750
Epoch 7/10
400/400 [==============================] - 230s 575ms/step - loss: 0.2560 - acc: 0.8969 - val_loss: 0.0935 - val_acc: 0.9825
Epoch 00007: val_acc improved from 0.97750 to 0.98250, saving model to simple1000-weights.07-0.09.h5
Epoch 8/10
400/400 [==============================] - 231s 577ms/step - loss: 0.2461 - acc: 0.9019 - val_loss: 0.0821 - val_acc: 0.9775
Epoch 00008: val_acc did not improve from 0.98250
Epoch 9/10
400/400 [==============================] - 231s 578ms/step - loss: 0.2606 - acc: 0.8981 - val_loss: 0.0722 - val_acc: 0.9825
Epoch 00009: val_acc did not improve from 0.98250
Epoch 10/10
400/400 [==============================] - 231s 578ms/step - loss: 0.2267 - acc: 0.9113 - val_loss: 0.1130 - val_acc: 0.9775
Epoch 00010: val_acc did not improve from 0.98250
Prediction & Submit
Prediction step
#prediction
nbr_test_samples=12500
#choose weights file manually
weights_path = 'simple1000-weights.07-0.09.h5'
test_data_dir = '../data/test/'
test_datagen = ImageDataGenerator(rescale=1./255)
test_generator = test_datagen.flow_from_directory(
test_data_dir,
target_size=(img_width, img_height),
batch_size=batch_size,
shuffle = False, # no shuffling, since filenames must match predictions. Shuffling may change file sequence
classes = None, #
class_mode = None)
test_image_list = test_generator.filenames
print('Loading model and weights')
predict_model = load_model(weights_path)
print('Begin to predict for testing data ...')
predictions = predict_model.predict_generator(test_generator, nbr_test_samples)
np.savetxt(session+'-predictions.txt', predictions) # store prediction matrix, for later analysis if necessary
Make submission file
Make submission file, format must match given sample_submission.csv
# submission
submission_file=session+'-submit.csv'
print('Begin to write submission file:'+submission_file)
f_submit = open(submission_file, 'w')
f_submit.write('id,labeln')
for i, image_name in enumerate(test_image_list):
basename=os.path.basename(image_name)
filename, fileext = os.path.splitext(basename)
prediction_class =predictions[i][0] # get predictions from array
f_submit.write('%s,%sn' % (filename, prediction_class))
f_submit.close()
print('Finished write submission file ..')
Submit the result to https://www.kaggle.com/c/dogs-vs-cats-redux-kernels-edition/leaderboard , click on “Late Submission”
We got score of 0.10979, still long way from the top (0.03) but not too bad for only 1000 samples.
Full source code for simple solution is available here: https://github.com/waskita/kaggle-dogs-cats/blob/master/simple-binary-classification.ipynb
Reference
- Tutorial on using Keras flow_from_directory and generators
- https://www.pyimagesearch.com/2017/12/11/image-classification-with-keras-and-deep-learning/
- http://blog.kaggle.com/2017/04/03/dogs-vs-cats-redux-playground-competition-winners-interview-bojan-tunguz/
- Code formatter: http://codeformatter.blogspot.com/